Recommendation Engines
Limited Time Offer!
For Less Than the Cost of a Starbucks Coffee, Access All DevOpsSchool Videos on YouTube Unlimitedly.
Master DevOps, SRE, DevSecOps Skills!
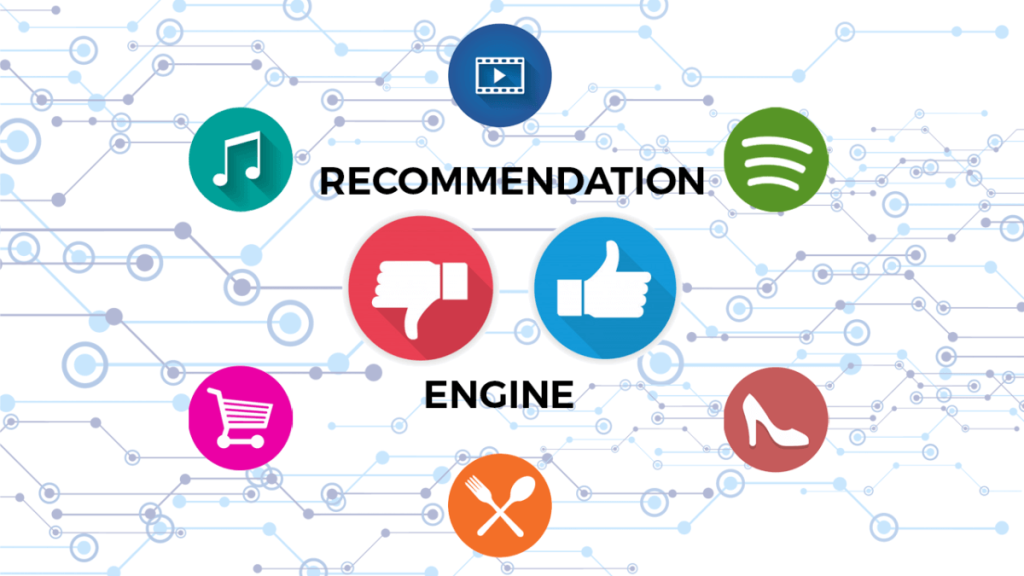
Have you ever wondered how Netflix recommends movies or how Amazon suggests products? The answer lies in the magic of recommendation engines. In today’s world, where we are bombarded with endless choices, recommendation engines have become an essential tool for businesses to provide personalized experiences to their customers.
What are Recommendation Engines?
Recommendation engines, also known as recommender systems, are algorithms that analyze user data to recommend products or services that they might like. These systems use machine learning techniques to predict user preferences based on their past behavior, interactions, and feedback.
There are mainly two types of recommendation engines: collaborative filtering and content-based filtering.
Collaborative Filtering
Collaborative filtering is a technique that uses the behavior of users to make recommendations. It works by finding similarities between users and suggesting products that similar users have liked in the past. For example, if User A and User B have liked similar products in the past, the system will recommend a product that User A has not yet seen but User B has liked.
Content-Based Filtering
Content-based filtering, on the other hand, focuses on the characteristics of the products themselves rather than the behavior of users. It works by analyzing the features of the products and recommending similar products to the ones that the user has liked in the past. For example, if a user has liked a romantic comedy movie, the system will recommend other romantic comedy movies based on the shared characteristics of the movies.
How Do Recommendation Engines Work?
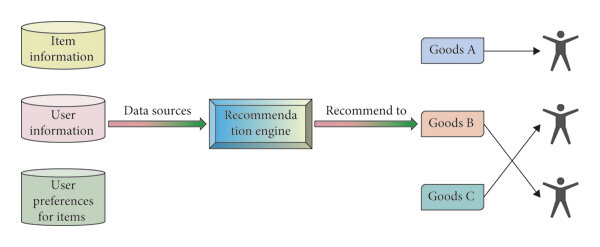
Recommendation engines work by collecting and analyzing data from various sources. These sources include user profiles, historical data, purchase history, and feedback. The data is then analyzed using machine learning algorithms to create a personalized recommendation for each user.
The recommendation engine starts by creating a user profile based on their behavior, interactions, and feedback. The engine then analyzes this data to identify patterns and similarities between users. Based on these patterns, the engine makes recommendations for products or services that the user might be interested in.
The recommendation engine continuously learns from user behavior and feedback to refine its recommendations. This process ensures that the recommendations remain relevant and personalized.
Benefits of Recommendation Engines
Recommendation engines provide several benefits to businesses and customers alike. Some of these benefits include:
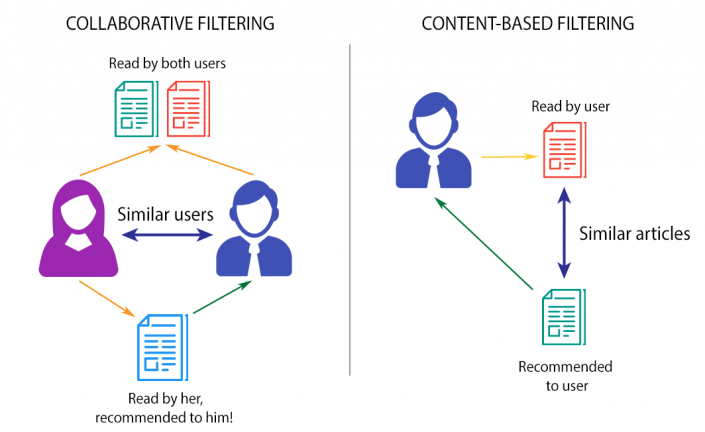
- Personalized experiences: Recommendation engines provide personalized experiences to customers by suggesting products or services that they might like based on their preferences and behavior.
- Increased customer engagement: Personalized experiences lead to increased customer engagement and loyalty.
- Increased sales: Recommendation engines can increase sales by suggesting products that customers are more likely to buy.
- Improved customer satisfaction: Personalized experiences lead to improved customer satisfaction as customers feel understood and valued.
Conclusion
Recommendation engines have become an essential tool for businesses to provide personalized experiences to their customers. These systems use machine learning algorithms to analyze user data and make recommendations based on their preferences and behavior. By providing personalized experiences, businesses can increase customer engagement, loyalty, and satisfaction, leading to increased sales.