The Pros and Cons of Google’s New AI Transparency Tools
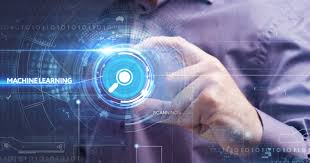
Limited Time Offer!
For Less Than the Cost of a Starbucks Coffee, Access All DevOpsSchool Videos on YouTube Unlimitedly.
Master DevOps, SRE, DevSecOps Skills!
Source:-insidebigdata.com
In this special guest feature, Jay Budzik, CTO at ZestFinance, discusses the set of developer tools that Google launched that allow data scientists to create explainable ML models, and also the reality of these new tools and how they are likely to impact the financial services market. ZestFinance is a software company that helps banks and lenders build, run, and monitor fully explainable machine learning underwriting models. As CTO, Jay oversees Zest’s product and engineering teams. His passion for inventing new technologies—particularly in data mining and AI—has played a central role throughout his career. Jay has a Ph.D. in computer science from Northwestern University.
Algorithmic models get a bad rap for being black boxes prone to unfair bias. They’re not—if you have the right tools to explain their decisions.
That’s why Google’s new suite of cloud-based explainable AI (XAI) tools is a step in the right direction for companies looking to adopt AI. These tools decipher, and defend, how specific data factors contribute to the output of machine learning (ML) models built atop complex neural networks.
While Google XAI should draw welcome attention and help bust a few ML myths, it likely won’t spur adoption for all companies—especially financial-services firms that need fully interpretable underwriting models to decide who can borrow and who can’t.
First, Google’s tools (now in beta) require customers to build and implement their models within the Google Cloud. But most companies need explainability tools that can be used in any cloud environment or on local servers.
Second, Google XAI isn’t flexible enough to accommodate more robust models. Like other explainability tools, Google’s are rooted in complex mathematical principles, including a version of the Aumann-Shapley method that uses a series of foils—called “counterfactuals”—to perfect an algorithm’s output. Andrew Moore, head of Google Cloud’s AI unit, described the process to the BBC in London last month: “The neural network asks itself, for example, ‘Suppose I hadn’t been able to look at the shirt color of the person walking into the store. Would that have changed my estimate of how quickly they were walking?’ By doing many counterfactuals, it gradually builds up a picture of what it is and isn’t paying attention to when it’s making a prediction.”
As compelling as that approach sounds, Google XAI won’t work with so-called ensembled models that weave together multiple models using diverse or competing techniques. “Ensembling” boosts the predictive power of AI credit scoring—assuming the collective system can be examined to assess how decisions are being made—and will become standard as lenders embrace ML over the next few years. For now, Google provides limited support for tree-based models and ensembles of trees and neural networks.
Third, while the Google AI “What-If” tool is pretty clever and allows modelers to test different scenarios at a glance, Google’s user interface could be tricky to use. Developers will have to learn a specific coding language and configuration convention to access explainability functions.
Last, Google’s explainability package is aimed primarily at data scientists—not credit analysts working for heavily regulated financial-services firms. In theory, data scientists at banks could build models using Google’s tools. But those same folks would need to build additional tools to test their models for accuracy and fairness and to generate all the compliance reporting required by regulators.
So while Google now offers a solid explainability tool for cloud-based neural networks, the vast majority of end-users will continue to demand more complete solutions that address their regulatory risk. Those solutions should include managed services (including expert help from a dedicated team), risk-management documentation (MRM) and model monitoring tailored for that industry, underwriting expertise and experience working with the largest lenders and, not least, a track record of deploying models that surpass regulatory scrutiny.
Google is taking important first steps to bring more transparency to the world of ML. That’s encouraging because the sooner our industry cracks open those scary black boxes, the better—for lenders, consumers, and the tech community.